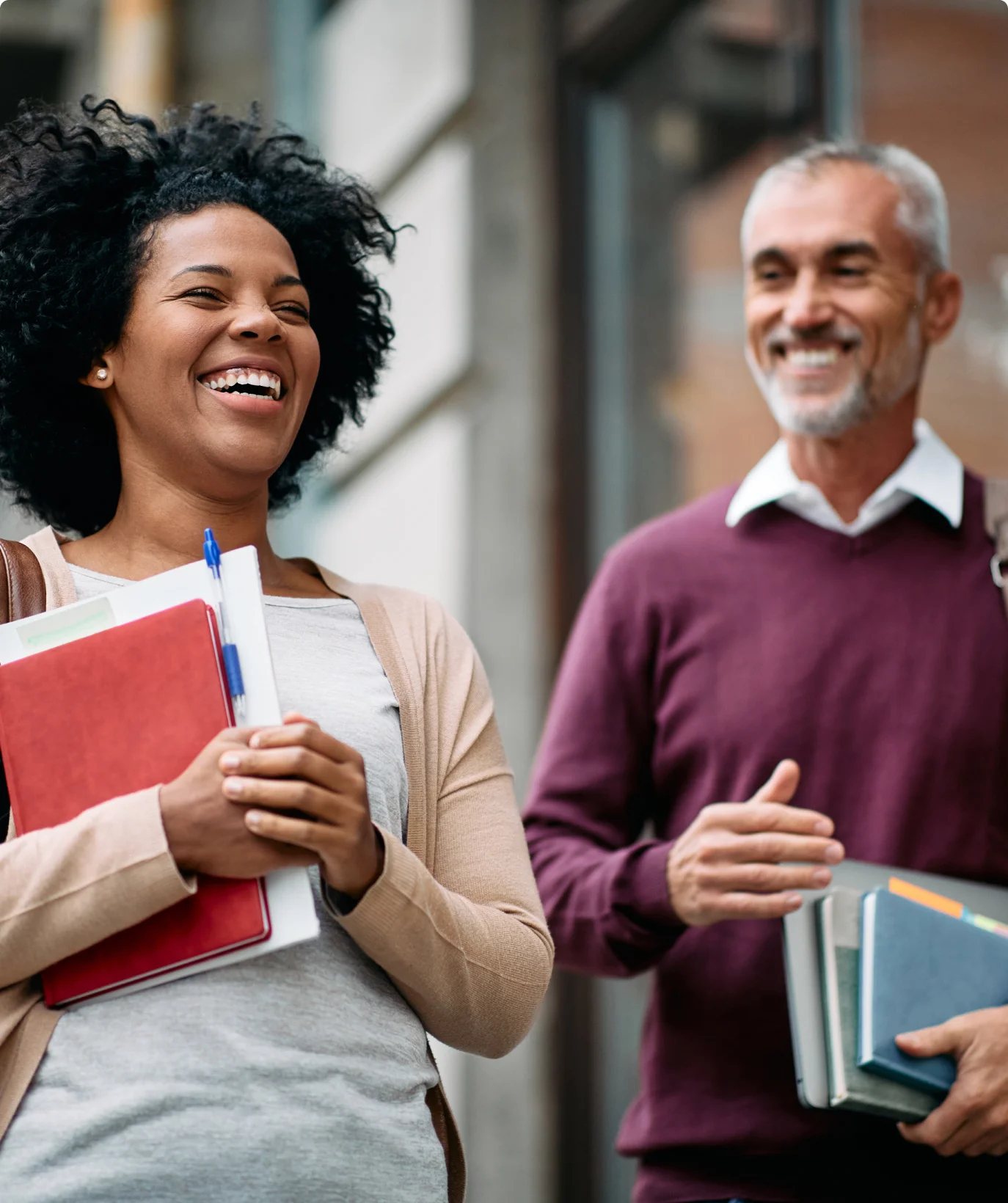
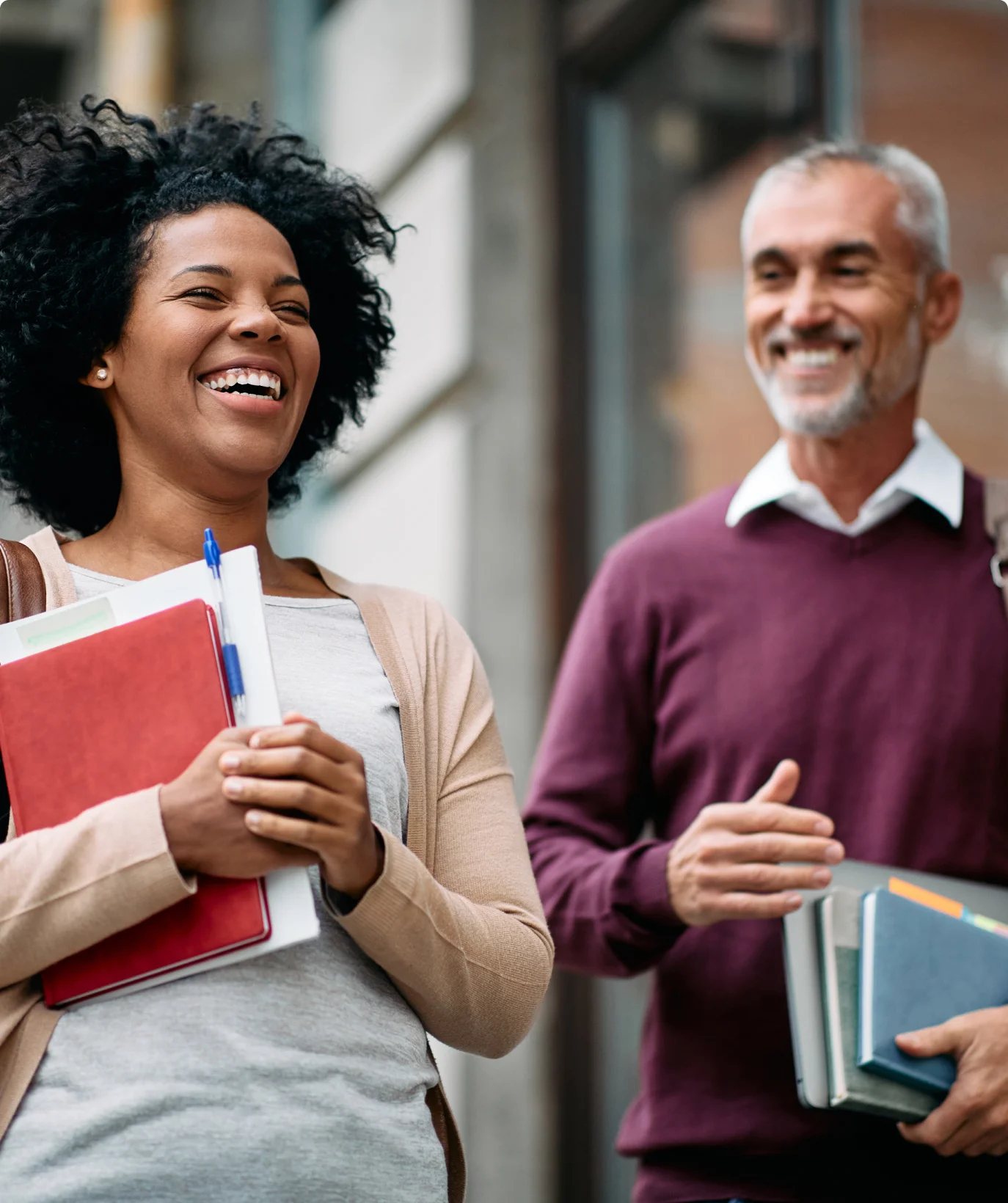
This course will explore the foundations of predictive analytical techniques on various data sets to guide the decision-making process. A variety of crucial topics will be addressed. Topics may include the following: (1) descriptive modeling techniques such as principal component analysis and clustering algorithms (i.e., k-means algorithm) and (2) predictive modeling techniques such as decision trees, linear regression, logistic regression and the k-Nearest Neighbor (k-NN). Other topics may be covered if time permits.
PREREQUISITE:
MGT6460 – Applied Business Analytics
COURSE COMPETENCIES:
UPON COMPLETION OF THE COURSE, THE STUDENT WILL BE COMPETENT IN: